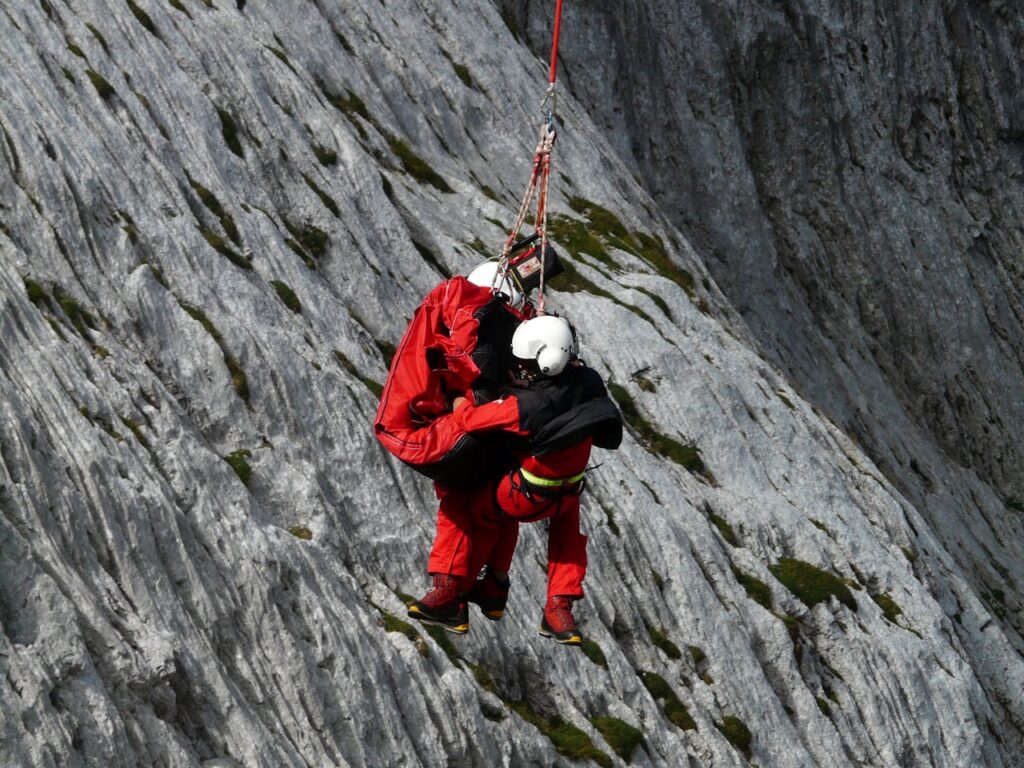
Risk Assessment and Management
Risk assessment and management have always been vital components of strategic planning, especially in industries where financial stability, safety, and long-term sustainability are paramount. Traditionally, risk management has relied heavily on historical data, human judgment, and statistical models to predict potential hazards and mitigate their impact. However, the emergence of Artificial Intelligence (AI) has revolutionized this domain by introducing new methodologies and tools that are far more accurate, efficient, and adaptive. AI has the potential to transform risk assessment and management, particularly in predicting potential losses and optimizing investment portfolios. This article explores the role of AI in these areas, analyzing real-world examples, discussing the implications for the future, and providing a critical analysis of the challenges and opportunities that lie ahead.
AI’s ability to process and analyze vast amounts of data at incredible speeds makes it an invaluable asset in risk assessment. Traditional methods of risk assessment often involve laborious data collection and analysis processes, which are not only time-consuming but also prone to human error. AI, on the other hand, can automate these processes, significantly reducing the time required to assess risks while increasing accuracy. For instance, in the financial sector, AI-driven algorithms can analyze market trends, economic indicators, and even social media sentiment to predict potential market downturns or identify emerging risks. This level of analysis is impossible for humans to achieve within the same timeframe, making AI an indispensable tool for modern risk management.
One of the most prominent examples of AI in risk management is its application in the insurance industry. Insurers have long relied on actuarial science to assess risk and determine premiums. However, AI has introduced a new dimension to this process by enabling more precise risk assessments based on a broader range of data points. For example, AI can analyze data from telematics devices installed in vehicles to assess a driver’s behavior and predict the likelihood of an accident. This allows insurers to offer personalized premiums based on individual risk profiles, rather than relying solely on generalized risk categories. This approach benefits both the insurer and the insured, as it leads to fairer pricing and encourages safer driving behavior.
AI is also being used to predict potential losses in various industries, particularly in finance and investments. In the stock market, for example, AI-driven algorithms can analyze historical data, market trends, and external factors such as geopolitical events or changes in regulatory policies to predict potential losses in investment portfolios. These predictions allow investors to make informed decisions about when to buy or sell assets, thereby optimizing their portfolios to minimize risk and maximize returns. AI’s predictive capabilities are not limited to the stock market; they can also be applied to other areas, such as real estate, commodities, and foreign exchange markets, providing investors with a comprehensive risk assessment across different asset classes.
A real-world example of AI’s impact on investment management is the rise of robo-advisors. These AI-driven platforms use algorithms to assess an individual’s risk tolerance, investment goals, and financial situation to create and manage a personalized investment portfolio. Robo-advisors continuously monitor and adjust the portfolio based on market conditions, ensuring that it remains aligned with the investor’s objectives. By automating the investment process, robo-advisors make it easier for individuals to invest in a diversified portfolio without the need for extensive financial knowledge or the assistance of a human financial advisor. This democratization of investment management has opened up opportunities for a broader range of people to participate in the financial markets, potentially leading to greater financial inclusion.
Despite the many advantages of using AI in risk assessment and management, there are also significant challenges and concerns that must be addressed. One of the primary concerns is the potential for bias in AI algorithms. AI systems are only as good as the data they are trained on, and if that data is biased or incomplete, the resulting risk assessments and predictions may also be biased. This is particularly concerning in areas such as lending or insurance, where biased risk assessments could lead to unfair treatment of certain groups of people. To mitigate this risk, it is essential for organizations to ensure that their AI systems are trained on diverse and representative data sets and to implement measures to detect and correct any biases that may arise.
Another challenge is the lack of transparency in AI-driven risk assessment models. Unlike traditional statistical models, which are often based on well-understood mathematical principles, AI models can be highly complex and difficult to interpret. This “black box” nature of AI can make it challenging for organizations to understand how certain risk assessments or predictions are made, leading to a lack of trust in the results. To address this issue, there is a growing emphasis on developing explainable AI models that provide greater transparency into how decisions are made. Explainable AI aims to make the inner workings of AI models more interpretable and understandable, thereby increasing trust and confidence in AI-driven risk assessments.
The use of AI in risk assessment and management also raises ethical concerns, particularly in relation to data privacy and security. AI systems often require access to large amounts of personal and sensitive data to make accurate predictions. This data can include financial information, health records, and even social media activity. While this data is invaluable for risk assessment, it also poses significant privacy risks if not handled properly. There have been numerous instances where data breaches have led to the unauthorized access and misuse of personal information. As AI continues to be integrated into risk management processes, organizations must prioritize data privacy and security by implementing robust data protection measures and adhering to relevant regulations, such as the General Data Protection Regulation (GDPR).
The future of AI in risk assessment and management is promising, with continued advancements in AI technology expected to further enhance its capabilities. One area of potential growth is the use of AI to assess and manage risks related to climate change. As the impacts of climate change become more pronounced, organizations are increasingly recognizing the need to assess and mitigate climate-related risks. AI can play a critical role in this process by analyzing environmental data, such as temperature patterns, sea levels, and carbon emissions, to predict the potential impacts of climate change on various industries and regions. For example, AI can help identify areas that are at high risk of flooding or wildfires, allowing governments and businesses to take proactive measures to mitigate these risks. Additionally, AI can be used to assess the financial risks associated with climate change, such as the potential for stranded assets in the energy sector or the impact of changing weather patterns on agriculture.
AI is also likely to play an increasingly important role in managing risks associated with cybersecurity. As cyber threats continue to evolve and become more sophisticated, traditional cybersecurity measures are often insufficient to protect against these risks. AI-driven cybersecurity tools can analyze vast amounts of data in real-time to detect and respond to potential threats, often before they can cause significant damage. For example, AI can be used to identify patterns of suspicious activity on a network, such as unauthorized access attempts or unusual data transfers, and take immediate action to prevent a breach. This proactive approach to cybersecurity is essential in a world where cyber threats are constantly changing and can have devastating consequences for businesses and individuals alike.
In addition to these specific applications, AI has the potential to fundamentally change the way organizations approach risk management. Traditional risk management often involves a reactive approach, where organizations respond to risks as they arise. However, AI enables a more proactive approach by predicting potential risks before they occur and allowing organizations to take preventative measures. This shift from reactive to proactive risk management has the potential to significantly reduce the impact of risks on organizations and improve overall resilience.
Moreover, the integration of AI into risk management processes can lead to more informed decision-making at all levels of an organization. By providing decision-makers with accurate, real-time risk assessments, AI can help organizations make better strategic decisions that align with their risk tolerance and long-term goals. This can be particularly valuable in industries where risk management is a critical component of success, such as finance, healthcare, and manufacturing. In these industries, the ability to accurately assess and manage risks can mean the difference between success and failure, making AI an invaluable tool for achieving long-term sustainability.
However, as AI continues to play an increasingly central role in risk management, it is important to recognize that AI is not a panacea. While AI can significantly enhance the accuracy and efficiency of risk assessments, it is not infallible. AI models are only as good as the data they are trained on, and there is always the potential for errors or biases to creep into the process. Additionally, AI-driven risk assessments should not be viewed as a replacement for human judgment. Instead, AI should be seen as a tool that can augment human decision-making by providing valuable insights and predictions that can inform risk management strategies. Organizations must strike the right balance between leveraging AI’s capabilities and relying on human expertise to ensure that risk management processes are both effective and ethical.
One critical area where AI’s role in risk management is still evolving is in dealing with complex, interdependent risks that span multiple domains. For example, the COVID-19 pandemic highlighted the interconnected nature of global risks, where a health crisis quickly escalated into an economic, social, and geopolitical challenge. AI’s ability to model complex systems and analyze vast amounts of data from diverse sources offers the potential to better understand and manage such multifaceted risks. However, this requires the development of more sophisticated AI models that can capture the nuances of these interdependencies and provide actionable insights across different domains.
Another area of ongoing research is the development of AI systems that can adapt to changing conditions in real-time. Traditional risk models often rely on static data, which can quickly become outdated in a rapidly changing environment. AI’s ability to learn and adapt over time offers the potential for more dynamic risk management processes that can adjust to new information and evolving risks. For example, in financial markets, AI-driven trading algorithms can continuously update their strategies based on real-time market data, allowing them to respond more effectively to changing market conditions. This adaptability is particularly valuable in today’s fast-paced, globalized world, where risks can emerge and evolve rapidly.
Looking to the future, the way forward for AI in risk assessment and management involves a combination of continued technological advancement and a commitment to ethical and responsible AI practices. As AI becomes more integrated into risk management processes, it is essential for organizations to invest in developing and